BW #100: Sports betting
Get better at: Cleaning data, grouping, and plotting
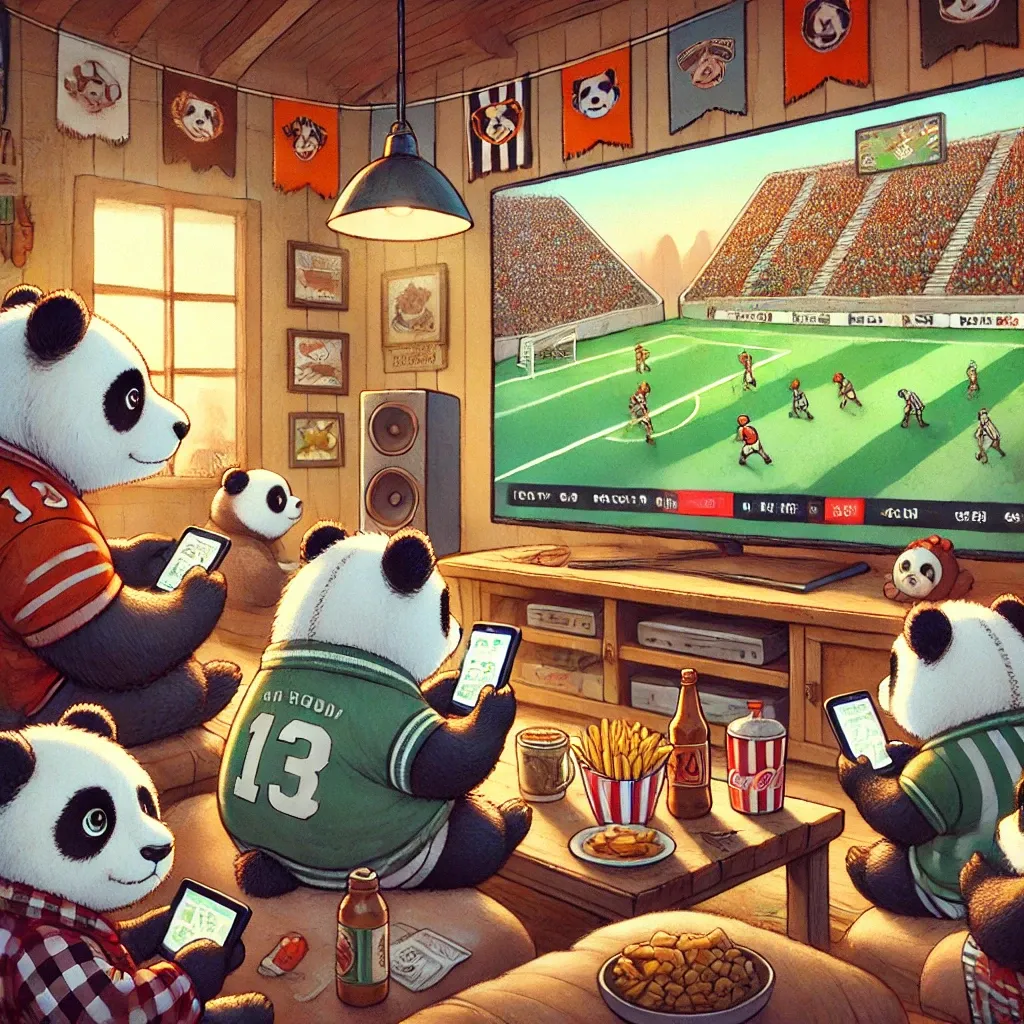
First and foremost: As you can see, this is Bamboo Weekly issue 100. I'm delighted to have reached this milestone, and appreciate everyone who has joined me over these first 100 weeks. I'm having a great time researching and writing this newsletter, and hope that you're enjoying solving these problems and improving your Pandas skills, one week at a time.
If you enjoy BW, then I'd appreciate it if you would spread the word to your Pandas-using friends and colleagues.
Second: Back in BW #60, I asked questions about Iceland, mostly because I had just spent 11 days on its famous Ring Road. I've written up a (long!) blog post about our trip, which you can read at https://lerner.co.il/2025/01/05/eleven-days-on-icelands-ring-road/ . Bottom line: It was amazing, and I can't recommend the trip enough.
And now, back to data analysis with Python and Pandas.
This week's topic is sports betting. I'll admit that I'm not into either sports or betting, but I've recently been listening to the latest season of Michael Lewis's "Against the rules" podcast (https://www.pushkin.fm/podcasts/against-the-rules), where he's discussing the changes that happened in American law that made it possible for people to bet on sports via their phones, as well as the societal impacts of those changes. I didn't expect to be interested in this topic, but I also didn't realize how widespread such betting has become, how much betting did (and still does) take place illegally, and just how high the societal costs are. And, of course, we've only gotten started.
Indeed, the Washington Post recently wrote an editorial describing the ills of betting on sports (https://www.washingtonpost.com/opinions/2024/12/29/sports-gambling-sportsbooks-bradley-act-betting/). The Economist ran a story on the topic a few weeks ago (https://www.economist.com/finance-and-economics/2024/12/05/how-sports-gambling-became-ubiquitous), as well as a "leader" piece saying how the growth in sports betting should be celebrated (https://www.economist.com/leaders/2024/12/05/americas-gambling-boom-should-be-celebrated-not-feared) -- followed by a gut-wrenching letter to the editor (https://www.economist.com/letters/2024/12/19/letters-to-the-editor) from a father whose son had committed suicide after his gambling debts piled up.
So, this topic has been in the news quite a bit lately. But what data can we find about it? The sports-betting companies aren't going to release more than some basic information. But at least in the US, sports betting is regulated by the individual states. (The podcast makes it clear that this regulation doesn't do a lot to prevent some real problems.) A number of states, in the interest of transparancy, publish data about sports betting. That's what we'll look at this week.
Data and six questions
One option for getting this week's data would have been to retrieve and parse it from a number of states. But Legal Sports Report (https://www.legalsportsreport.com/) is a Web site that (according to their home page) "covers the legal online sports wagering industry, including sports betting and daily fantasy sports." And getting the data from one site is almost certainly going to be easier than getting it from multiple sites with different reporting standards. (Don't worry; there's still room for some challenges here!)
LSR has a page (https://www.legalsportsreport.com/sports-betting/revenue) breaking down the amounts spent on sports betting across all states, across all months, and then for each month in each state. Each table has several columns:
- Market or month
- Handle, meaning the total amount of money that was bet.
- Revenue, meaning how much money the sports-better company kept after paying out winning bets. You can think of this as the company's gross income. (Note that revenue is only calculated after paying people who made winning bets.)
- Hold, the amount of bet money (handle) that the company kept (revenue), expressed as a percentage. So if all of the bets, on all sports, were \$1,000, and the company paid out \$900 to all of the people who won bets, then we would say that the handle was \$1,000, the revenue was \$100, and the hold was 10%.
- Taxes, how much the sports-betting company paid in taxes.
Note that the LSR site doesn't have data for all states. You'll only need to retrieve and work with data from the following state abbreviations:
AZ AK CO CT DE DC IL IN IA KS KY LA ME MD MA MI MS MT NV NH NJ NY NC OH OR PA RI SD TN VT VA WV WY
Here are six tasks and questions for you this week. The learning goals include cleaning data, grouping, and plotting.
I'll be back tomorrow with my full solutions and explanations, as well the Jupyter notebook I used to solve these problems.
- You cannot use
requests
orpd.read_html
to retrieve the page from LSR. Rather, you'll need to go there with your browser, save the HTML ,and then usepd.read_html
on that saved HTML file. Do so, creating a single data frame in which the columns aremarket
(i.e., the state's name or abbreviation), along with the month, handle, revenue, hold, and taxes from the LSM site. Treat?
as aNaN
value. Also remove the $ from the start of dollar columns, commas (,) from integer columns, and % from the Hold column. Remove rows in which theMonth
column contains the string "Total". - Remove
NaN
values. Turn theMonth
column into a datetime, turn the handle, revenue, and taxes into integers, and turn the hold into a float.